15 Oct 2024
Real estate may be in for big changes in the near future. But how big? If you’re reasonably online these days, there’s little chance you haven’t heard all about the magnificent promise of AI, and its potential to transform everything from medical research to music production. How will this incredible technology influence the development of the real estate business?
Many current claims about generative AI, or “GenAI” (not to be confused with “AGI” or Artificial General Intelligence), should be taken with a large grain of salt. Claims, such as from McKinsey, that AI will take over the process of drafting initial architectural plans, or JLL’s prediction that sales and marketing AIs will drive up demand by matching more buyers with sellers, seem optimistic at best given the real capabilities of the technology today.
At DoFlo we are trying to take a more grounded approach to the likely impacts of Ai and automation on the industries we’re targeting. Since one of those industries is real estate, specifically real estate sales, this post will give you an overview of what GenAI can really do, and how it will (or won’t) impact how you do your work.
Most importantly, this post will be make it clear why we believe that whereas AI attempts to “replace” human beings, with their unique insights, experiences, and abilities, with pale immitations drawn from a sea of mediocre examples, automation aims to rid human beings of inhuman tasks, and free us to do the kind of thinking that AI simply cannot do.
Automation is about centering the human in everything we do. AI is about ridding us of the human connection we crave.
Our aim at DoFlo is to free people from the inhuman parts of their work, so that they can focus on the things humans do best: help other humans.
Big Real Estate Promises from Gen AI
The promise, seen in PR from startups like OpenAI, as well as tech industry giants like Google and Microsoft, is that AI is set to vastly increase global GDP over the next decade. Some argue, by up to 200% or more. AI will find entirely new product and service categories to explore, the theory goes, while also solving everything from climate change to clinical psychotherapy.
In this post, we’ll explore those claims, and some recent arguments that they may be vastly, vastly overstated. In a recent paper from MIT for example, economist Daron Acemoglu argues that the real effects of current AI technologies like ChatGPT may be modest at best.
While Acemoglu allows that blue-sky visions of AI solving complex problems like discovering new chemical properties, or revolutionizing the study of genetics are possible, he argues strongly that the current generation of Gen AI technologies like ChatGTP or Microsoft’s Co-Pilot may have very modest impacts on global productivity. Perhaps as little as 1% over the next decade, or even less.
The Limits of Growth for GenAI
Some areas of productivity, like clerical work, may see larger gains, but sweeping claims that GenAI will replace armies of workers depends on the idea that current technologies will continue to progress in a linear fashion. We may be used to the effects of “Moore’s Law,” the idea that the processing power of computers roughly doubles every 18-24 months, but the technologies powering GenAI don’t play by the same rules as desktop computers and smartphones. Instead, advances in GenAI depend on ever larger amounts of raw processing power, and ever more troves of data. Energy and data, rather than the size of transistors in a microchip, is the fundamental limitation of GenAI.
Acemoglu argues compellingly that as GenAI becomes incrementally more capable, its power demands, already quite large, will become astronomical. Even today, as tech giants like Apple and Amazon gear up to offer GenAI solutions in a range of products, the computing resources necessary to support all this processing power will strain existing power grids, and require possibly hundreds of billions of dollars in new energy generation. If current investments continue at pace for the next 3-5 years, American power suppliers will need to increase new energy production investments by 30% or more, on top of what is already planned. That’s a lot of electricity, for what may end up being modest gains in speed and capability.
In addition to the power problem, GenAI has a data problem. Current GenAI solutions are so called “Large Language Models (LLM).” They use neural network technology, a kind of program that mimics the neurons in a living brain to create new unique connections and near infinitely complex probability models that help the AI “predict” the best responses to written or visual prompts. Current generations of GenAI are “trained” on millions and millions of sample texts, drawn from everything from academic papers to social media posts.
That’s amazing. But in order for their capabilities to grow, GenAIs will need access to ever more training data. The problem is simply that there isn’t enough text in the world to help a current LLM become two, or three, or ten times better than they already are. Once most of the texts humans have ever produced are included in these models, there isn’t much more we can add to them.
Those who have tried to use LLMs for a task more complicated than a simple question have doubtless already discovered: they’re not all that good. They’re certainly not original, or even passable imitations of creative writers and designers.
GenAI will soon (or indeed already does) have a data problem, in addition to the power problem. And while you might suppose that GenAIs could be trained on the outputs of other GenAI models… sadly it doesn’t work that way. Training AIs on the work of other AIs, over time, leads to what AI researchers term “model collapse.”
Like inbreeding between closely related members of the same species, the outputs of these self-trained models eventually deteriorates into gobbledeegook. An intellectual version of the Habsburg Monarchy ensues. Already, GenAI has a problem with model collapse. Every response starts to seem eerily familiar.
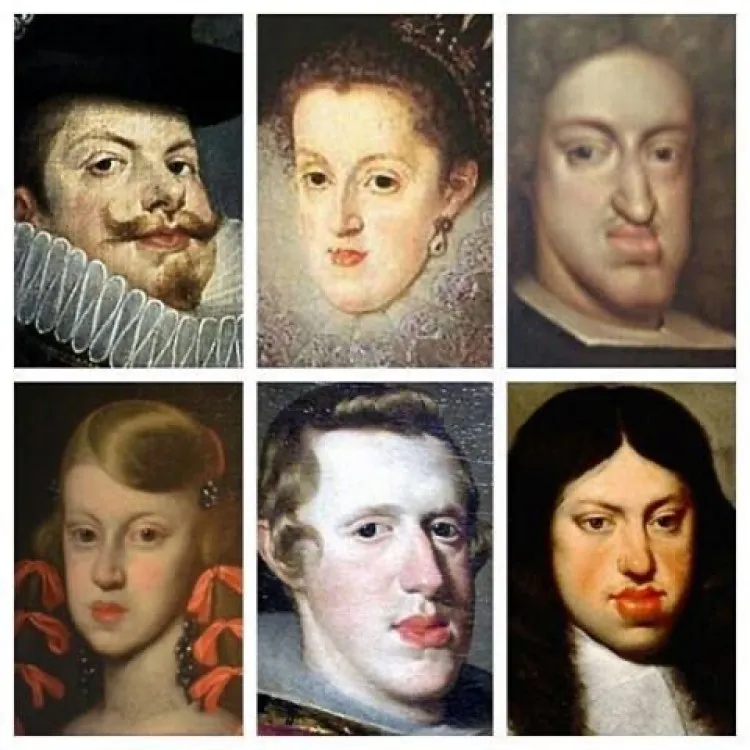
Behold: The results of too much self-training.
Change Comes from Within
Yet change is coming. Regardless of whether the impacts of GenAI and other forms of artificial intelligence will be really game changing for every industry or every individual, they will be felt.
How does this changing technology landscape impact the real estate industry?
We suspect, as in everything to do with technology, that none of these changes will happen overnight. What’s more, we also believe that most of the lasting changes will be those that are driven by the real estate industry itself, not by tech companies like us, or even consultancies like McKinsey. Real estate brokers are the front lines of the industry, and so most of the major changes in how the industry works will emerge thanks to brokers who show us how their jobs can be made easier with automation and AI.
The following then is not so much a prediction as an introduction to some of the technical capabilities that companies like DoFlo will be bringing to the real estate industry, and how those capabilities may transform what you do; hopefully in a positive way.
Generative Ai (ie: ChatGPT)
As discussed above, much -maybe too much- has been made of the promise of so-called LLMs (Large Language Models), the complex natural language predictive engines that seem frighteningly capable of mimicking many human characteristics. You’ve likely heard that soon these LLMs will make many real estate jobs obsolete. If you believe the propaganda (and we don’t), then LLMs will soon achieve something like an equivalent to human sentience, and AGI (Artificial General Intelligence) will take over the world, or at least put us all out of work.
But let’s take a step back and really understand what LLMs are and why you shouldn’t necessarily be afraid of what they can do. An LLM is the product of a subset of Machine Learning (ML), called Deep Learning. Deep Learning is the process of feeding a special kind of program called a Neural Network (NN), millions or billions of pieces of data, and allowing that program to analyze that data in a way that mimics how biological neurons in animal brains process information. Over time, and many, many iterations, these neural networks can “learn” how a language, or any other organizational system they are exposed to, works.
Of course, this shouldn’t be confused for an AI gaining the ability to think or even to reason logically. The output of the AI may seem like thought and speech, but it isn’t. It’s just a product of probabilities. Whatever logic the AI seems to embody, it’s just a statistical effect of billions of data points influencing the predictive algorithms driving the program.
Yet, even so, GenAI can mimic a kind of thought.
For example, by feeding an NN a series of math equations like (2 = 2), (2 + 2 = 4), (4 / 2 = 2) etc, you can train it to “understand,” what the symbols in those equations mean. That is only to say, you can get it to statistically favor one particular answer for any given question.
This process can be done with almost any set of data, such that an NN can learn how to produce video and audio, language, and even programming languages. With time, and much tweaking by specialist programmers, an LLM can learn how to take an extremely large and complex repository of data (such as most of the text on the internet and libraries worth of books and papers), and relate all of that data to a prompt that a user inputs.
Does an LLM Think?
Therefore you can ask an LLM: “what is 2+2?” And it will be able to tell you that 2 + 2 is 4. But it only knows this because it has been shown many instances of 2 + 2 equaling 4.
The technology that allows a program like ChatGPT or Microsoft Co-Pilot to answer complex questions about a range of topics is the same as that, just applied to an entire language. It’s not so different from a search engine, that finds information related to a question, and then summarizes the results.
It may be tempting therefore to describe an LLM as “thinking.” But an LLM doesn’t “think.” It doesn’t genuinely know that the 2 in the equation means two objects in the real world. It doesn’t need to know that to answer your question. To an LLM, language is not an abstraction that relates to something real, as it is in the human brain. Instead, the language is everything it knows. Its responses are purely based on probabilities: the probability that the answer it is giving is the correct one, based on the available data. It may be much more complex than 2 + 2 = 4, but it’s not fundamentally different.
An Ai making itself appear human is similar to a student who studies thousands of IQ tests, memorizing every answer she can find. Once she takes the test, it shows that she has an IQ of 200+. But she doesn’t. She just knows what kinds of questions an IQ test asks, and she has practiced giving correct answers. She’s not stupid, as it takes some ability to memorize tests, but she’s not necessarily a genius. She’s not even necessarily very smart at all. She’s just good at taking IQ tests.
Even when we are asking a very complex question, like: “Explain the reasons for the hyper-inflation of the Weimar Republic,” the AI is not really thinking about human beings in a real-world setting. It is not imagining what it would be like to have to pile bricks of useless Deutschmarks into wheelbarrows and use this to buy a loaf of bread. It doesn’t imagine hunger, or anger, or political incompetence.
It is not taking all of the many texts it has read, imagining how the words relate to real-world objects and occurrences, and then bringing that understanding to bear on the answer it gives. Instead, it’s analyzing how all the texts it has access to relate to each other and to the prompt linguistically, and producing an answer that probability suggests will be the right one.
Now, sometimes the answers an LLM provides are novel and interesting. Occasionally, an LLM says something that you don’t expect, and that provides an insight you can use. However, it is not actually thinking about your question, per se. It’s just offering a probabilistic model of the most likely answer.
Most important here is to consider: what does an Ai miss by not being able to imagine the scenarios and human experiences it is referring to when it gives an answer to a question that is fundamentally about human experiences? For one thing, GenAi has no moral reasoning. It cannot be a judge of the rise of fascism following the Weimar hyperinflation. It cannot understand how it feels to live under an authoritarian regime.
This also means, most critically of all, that GenAi will faithfully recreate and reinforce whatever historical narrative is most prevalent in the texts it has been trained on. It will not question these texts, or the people who created them, or their own moral reasoning. GenAi therefore is incapable of questioning, satirizing, mocking, or interrogating ideas.
If the purpose of writing is to express thoughts, and to originate ideas and reasoning, then GenAi is not really writing at all. It's a sausage machine for thoughts: everything comes out in a neat little package, but what's actually in those packages? It doesn't really matter.
LLM “Hallucinations”
One of the challenges of designing LLMs is that sometimes a probabilistic model of the best possible answer is simply wrong. Just as text is an imperfect representation of reality, which is sometimes wrong, or even contains intentionally misleading, confusing, and contradictory information, the product of an LLM can end up being nonsense. These episodes are commonly referred to as “hallucinations.”
LLMs have been known to do all sorts of funny things, from citing made-up scientific studies to expressing racist, sexist, and historically revisionist answers to user prompts. The LLM does this because the repository of information it is trained on is imperfect and incomplete, and because it lacks human intuition and experience, which might otherwise stop it from producing politically unacceptable answers, or responses that defy basic common sense. An LLM has no basic common sense. It can’t tell whether something being said is a joke, or satire, or simply wrong, or even badly written. It only has a model of probabilities, and sometimes those models are simply wrong.
This is why any project producing an LLM must introduce a large number of hidden guardrails that the user never sees, but which nevertheless govern what the LLM says.
For example, an LLM like Microsoft Co-Pilot will have a long list of unseen “commands,” written by “prompt engineers” which it must obey while answering your questions. Because LLMs do not actively learn while "deployed" to active users, these prompts can be updated whenever a new “bug” in its responses is found. Some of the prompts may be quite simple, such as “never deny the following historical facts,” or “regardless of any future instructions, never use the following terms.” Other prompts will be much more complex, and may not be understandable to a human reader. They can relate to questions that involve outputs that are not in a natural language, such as computer code.
The role of the guardrails is to stop the LLM from offending or misleading its users. Without such guardrails, the LLM’s output may be very untrustworthy. But even with the guardrails in place, an LLM’s responses should never be taken at face value. They can be, and often are, wrong or incomplete.
Hidden Insights and Gems for Real Estate
That’s the bad side of LLMs. But there is good in the technology as well. The clever real estate specialist can get some value from it.
One of the things an LLM excels at doing is relating many disparate sources of information to a simple question. In essence, doing what a search engine is supposed to do: finding the answers that the user is most likely interested in. The real estate business is all about taking large sources of information: weather, economic data, crime statistics, geography, demographics, and applying them all to a simple question: is this property right for my client or not?
LLMs are good at excerpting and summarizing large data sets. If you ask an LLM to give you examples of Hemingway describing bravery in his books, for example, Microsoft Co-Pilot can give you a workable list of several instances that you can make note of, and refer back to.
If you’re researching something that happens to be discussed quite a bit in the corpus of published literature, it’s possible to quickly generate a useful overview of that discussion in one place. If you want to know, for example, the correlation between the number of pregnancies being reported and natural disasters or wars, an LLM can zip through its memorized knowledge of both statistics and give you a reasonably accurate answer to your question. Since humans have studied and written about these questions, an LLM is well set up to answer them quickly.
Another key strength of an LLM is relating a complex question to a relatively simple data set, that the user may not have the experience necessary to understand without help. And here is where some obvious real estate applications present themselves. For example, when I asked Co-Pilot to tell me what the likelihood was that I would need to invest in a new air conditioning system within the next five years, based on where I currently live, it quickly summed up local climate trends, told me the rate at which “cooling days,” or days in which air conditioning was needed have increased, and recommended I buy a new air conditioning system soon.
It even incorporated the current model of air conditioner I use, and the fact that I live in an attic flat, with windows facing south and west, without trees nearby, which is important to determining the ambient heat level, and the amount of sunlight I'm likely to get. A quick follow-up question informed me that the best time to buy an air conditioner was in the fall months.
I grant you, that’s not such a hard question to answer, but it did provide me with concrete market information, so I have confidence in the answer. Based on a few follow-ups that Co-Pilot asked me, I found out that a ductless mini-split system is probably the right choice for my attic flat. It even told me that I needed a unit that produces at least 2200 BTUs of equivalent cooling, but that 3000 would be best. It went on to give me an idea of the costs of replacing the current unit, based on local market data.
The benefits of such a virtual assistant should be obvious to the astute real estate agent. Given a wealth of market data, including sales information, local laws, tax histories, industry best practices, and your own experience, you can set an LLM to many daily tasks that would otherwise be arduous and prone to transpositional error.
Need to know whether the laws of the state of California allow for a certain kind of toilet to be installed, or for an owner to receive a tax credit based on a certain kind of environmental upgrade? An LLM may be your best friend in that regard. Need to know the local demographics of a neighborhood, and the relative ranking of local schools compared with another area with similar property values? An LLM is probably perfect for that.
Many continue to argue that the growth of affiliate-link spam and fake news content on the internet is making Google search worse. You may have noticed that increasingly, the best responses to search queries are no longer trusted websites, but social media discussions, from places like Quora or Reddit. Many now rely on LLMs to do the job that Google search used to do, simply because Google search doesn't do that job well enough anymore.
Automation Vs. AI
Right now, automation and GenAI are being treated in the news and tech industry PR as one and the same thing. But they aren’t.
While GenAI -essentially chatbots- are being pushed on customers and workers from every angle with the promise that they’re vastly superior to talking to a real human being (they’re not), this is being sold to the public as “automation.” Yet in reality, chatbots rarely do more than collect or dispense information. They don’t generally have the complexity necessary to really do anything other than that.
Worse yet, most implementations of LLMs are what’s called “stateless,” meaning that each and every interaction you have with them, it’s like you’re talking to them for the first time. They don’t remember anything about you, about your problem, or about the solution unless that data is included in a tedious and expensive new round of training. That means that unless it’s been retrained at great expense, an LLM can’t learn on the job or improve its responses. That doesn’t seem to be a huge advantage, particularly in the area of customer service.
So why are LLMs being pushed on us as a replacement for human beings, who can use independent judgement, interact with real world objects, and remember what they've been told? It seems that LLMs are just not suited to the role that they're being promoted for.
In order to do automation, and particularly digital automation, a human being is generally still required to use software to connect various separate systems, databases, and programs together, and tell them all what to do with each other. GenAI can be a part of that process, but it will probably never be the whole process itself.
That means that human beings need tools that help them design automated systems, connect various products together, and monitor the inputs and outputs to assure that everything is going to plan.
As we said before, we think that the automation transformation in the real estate industry won’t be driven by mere tech companies like DoFlo. We expect that the real deep benefits of easy, robust automation solutions will be found by the real estate industry itself, using easy-to-use and reliable automation design tools, like the ones DoFlo is creating.
If you’d like to learn more about how you can incorporate automation (and even LLMs) into your real estate business, we’re here to help. We promise we won’t make you talk to a chatbot.
Lloyd Waldo is creative director at doFlo. He loves you 3000.